Governance-First AI in Healthcare: Balancing Innovation and Ethics
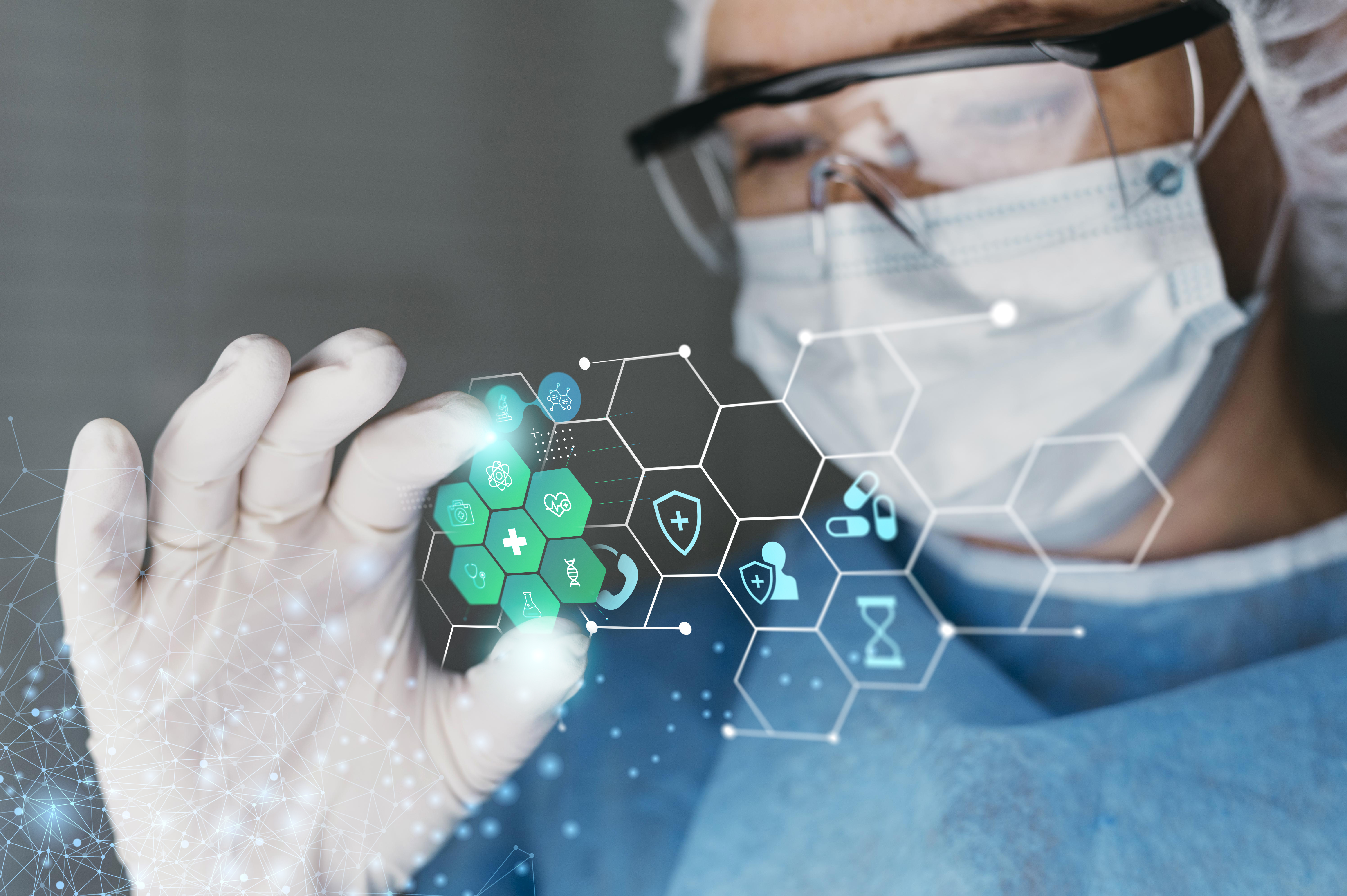
Discussion with Brian M. Green
The integration of artificial intelligence (AI) into healthcare presents vast opportunities, yet it also demands rigorous ethical oversight and governance structures. With over 30 years of experience in healthcare and pharma brands, Brian M. Green has navigated the complexities of public health research, digital health, and AI-driven patient-centered solutions. His extensive background spans healthcare quality improvement initiatives, data governance and privacy in online patient communities, and AI governance and use-case optimization. In this article, we explore how healthcare organizations can bridge the gap between AI innovation and responsible implementation, focusing on readiness assessments, ethical frameworks, patient-centric approaches, bias mitigation, and leadership in AI governance.
Aligning AI Use Cases with Real-World Applications
One of the primary challenges healthcare organizations face is translating AI innovation into clinical practice. While AI-driven tools promise enhancements in diagnostics, workflow efficiency, and patient monitoring, their success depends on well-defined use cases and seamless integration within existing medical workflows.
"Healthcare providers need to ensure that AI solutions fit into existing workflows. If physicians and staff don't use a tool because it doesn’t integrate well, its potential benefits are lost". Green notes that AI solutions must be developed with a deep understanding of the clinical environment. A diagnostic AI tool may be promising, but if it does not align with physician workflows or is difficult to use, it will fail to deliver value.
The first step is conducting a readiness assessment that evaluates an organization’s preparedness for AI adoption. This involves aligning AI use cases with strategic business goals, assessing data infrastructure, workforce AI literacy, and ensuring compatibility with clinical workflows. Many AI solutions falter when they fail to consider real-world clinical operations and the human element. For example, remote patient monitoring must accommodate the daily needs of both patients and caregivers, ensuring seamless data collection and interpretation, and providing that data to care teams in ways that link to their ongoing responsibilities.
Additionally, aligning AI solutions with patient engagement objectives is crucial. Healthcare AI tools must enhance operational efficiency and empower patients to manage their care more effectively with their daily needs. Whether through improved access to specialists, timely diagnostics, or tailored treatment recommendations, or automated alerts for provider triage decisions, AI must prioritize meaningful patient outcomes.
Ethical AI Governance: A Structured Approach
Ensuring AI aligns with ethical and responsible practices requires a structured governance framework. Organizations must move beyond superficial regulatory compliance and embrace a governance-first approach that embeds ethical considerations from the outset. "A governance-first approach to AI ensures that ethical considerations are not an afterthought but a fundamental component of development and deployment”, Green emphasizes. Without a structured governance strategy, organizations risk deploying AI solutions that introduce unintended biases, compliance issues, and ethical concerns.
A robust AI governance framework begins with a comprehensive assessment of key domains: data management and risk mitigation, leadership oversight, operational planning, workforce readiness, and cybersecurity. Green advocates for a multistakeholder approach that includes legal, compliance, IT, clinical experts, and patient advocates, ensuring a holistic strategy for AI adoption.
Governance structures must be iterative, adapting to evolving AI capabilities and regulatory landscapes. Organizations should establish multidisciplinary AI governance committees that meet regularly to review model performance, assess risks, and refine ethical guidelines. Green stresses that governance is not a one-time implementation but an ongoing process that requires continuous monitoring and refinement.
Patient-Centric AI: Ethical Implementation for Maximum Impact
A truly ethical AI framework in healthcare must prioritize patient needs. AI should not only enhance clinical efficiency but also improve patient outcomes and engagement.
"Patient involvement should not be sporadic—it must be integrated throughout the AI lifecycle to ensure that solutions address real needs and remain ethical in practice and outcomes". Green notes that healthcare AI solutions often fail when they do not account for real patient experiences. Simply adding patient feedback late in the development process is insufficient; instead, patients should be engaged from the ideation phase through post-deployment monitoring and evaluation.
Traditional AI development often involves patient input at select stages, such as testing and validation. However, Green argues that patient insights should guide the entire AI lifecycle, from ideation to deployment and evaluation. By involving patient advocacy groups and caregivers in governance discussions and prioritization, organizations can ensure that AI-driven healthcare solutions address diverse patient needs.
Healthcare institutions can draw inspiration from clinical research ethics by integrating patient representatives into AI oversight committees. This approach aligns AI governance with the principles of Institutional Review Boards (IRBs), ensuring that AI tools respect patient rights, privacy, and consent. Furthermore, AI implementations should be continuously monitored for their impact on patient care, refining algorithms based on real-world feedback.
Addressing Bias and Ensuring Equitable AI Solutions
AI bias remains a critical challenge, particularly in healthcare applications where disparities in training data can lead to inequitable patient outcomes. Bias in AI models is often a reflection of historical inequities in healthcare data, making proactive bias mitigation essential.
"Bias isn’t just about flawed algorithms—it’s about ensuring AI models are trained on diverse and representative data to avoid systemic disparities". Green emphasizes that addressing bias requires more than refining algorithms—it demands a commitment to collecting and integrating diverse datasets, and committing to continuous model evaluation.
Mitigating bias requires a multifaceted approach. First, organizations must assess their data sources to identify gaps and imbalances. AI models should be trained using diverse datasets that account for variations in race, gender, socioeconomic status, and rare diseases, for example. Additionally, synthetic data generation and digital twin models that are adequately validated can help fill gaps in underrepresented patient populations.
Green stresses that bias mitigation is not a one-time effort but an ongoing process. AI models must be continuously evaluated and refined to ensure they remain equitable as patient demographics and medical knowledge evolve. Organizations must establish AI oversight mechanisms that incorporate real-time monitoring and bias audits to identify and correct disparities proactively.
Leadership’s Role in AI Governance
Strong leadership is vital in embedding ethical AI practices within healthcare organizations. While AI governance frameworks provide structure, leadership commitment ensures that governance translates into action.
"AI governance isn’t just a legal or compliance function—it requires leadership to drive cultural change and strategic alignment", Green underscores. AI governance is not an isolated initiative but must be championed by leadership at all levels.
Many healthcare institutions struggle to determine where AI leadership should reside. Historically, AI initiatives have fallen under the purview of Chief Technology Officers (CTOs) or Chief Information Officers (CIOs). However, Green argues that AI ethics should have dedicated leadership, such as a Chief AI Ethics Officer, to ensure that ethical considerations remain a core focus.
Leadership must champion AI literacy across the organization, providing ongoing workforce training and fostering a culture of responsible AI use. Additionally, executives must allocate adequate resources to AI governance, ensuring that AI oversight committees have the necessary support to function effectively.
Key Takeaways and Conclusion
AI presents transformative opportunities for healthcare, but its success depends on a governance-first approach that integrates ethical considerations at every stage. Brian M. Green’s expertise highlights several key takeaways for organizations looking to implement AI responsibly.
"A governance-first approach ensures that AI is not just implemented efficiently, but ethically, protecting both patients and organizations from potential risks”, Green emphasizes: Organizations must strategically integrate AI governance from the outset to avoid compliance and ethical pitfalls.
First, readiness assessments are essential for aligning AI solutions with real-world applications. AI tools must seamlessly fit into existing workflows and directly contribute to improved patient outcomes. Second, ethical AI governance requires continuous evaluation of risks, biases, and compliance. AI oversight should be an ongoing process, supported by leadership and governance committees.
"Bias in AI isn't just a technical flaw—it has real-world consequences for patient safety and equitable access to care”. Green highlights the need for diverse training datasets and continuous bias evaluation to ensure fair and equitable AI-driven healthcare.
Finally, leadership plays a crucial role in responsible AI implementation. Organizations must foster AI literacy, allocate resources for governance, and embed responsible AI practices into their culture. Green notes that “Organizations prioritizing AI governance will be better positioned to navigate regulatory complexities, enhance patient trust, and drive long-term innovation in the healthcare sector.”